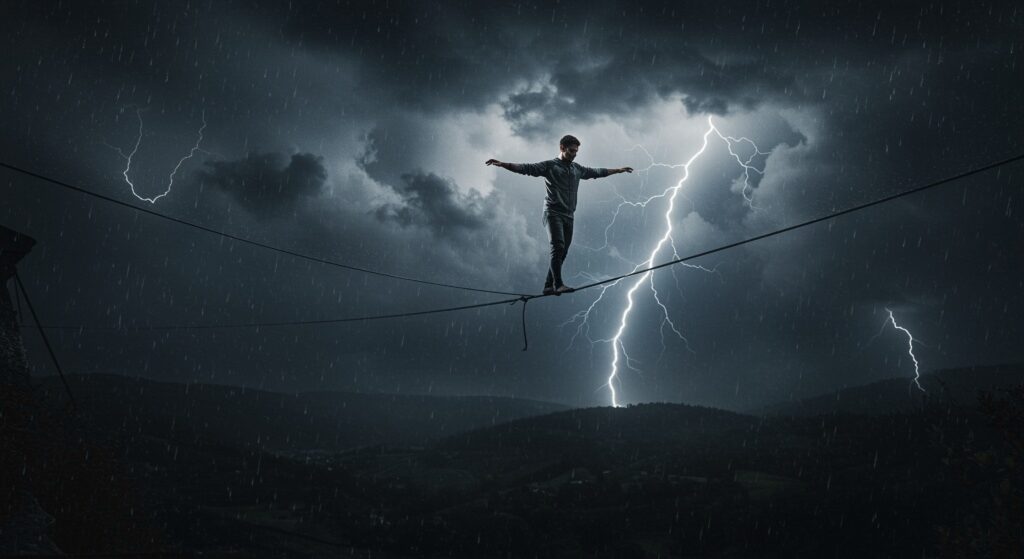
Macro-aware risk parity
Risk parity is an investment strategy that allocates risk exposure equally across asset types through volatility-based calibration and leverage. A most profitable risk parity strategy in the past decades has been the equity-duration “long-long”, which harvests combined equity and long fixed-income risk premia, while containing return volatility through diversification. Alas, this position is vulnerable to “autonomous” monetary tightening shocks, i.e., surges in real interest rates without commensurate economic improvements.
The probability of such downside shocks depends on the macroeconomic environment. Hence, by adapting volatility-targeted risk-parity positions to economic overheating measures, strategies become macro-aware and more information-efficient. We show that simple point-in-time macro scores of excess inflation, growth, and overconfidence have predicted equity-duration returns across eight developed markets and for over three decades. Macro-aware signals have materially outperformed simple volatility-targeted positions, both in terms of absolute and risk-adjusted returns. The long-term effects of macro awareness on cumulative net asset value have been huge.