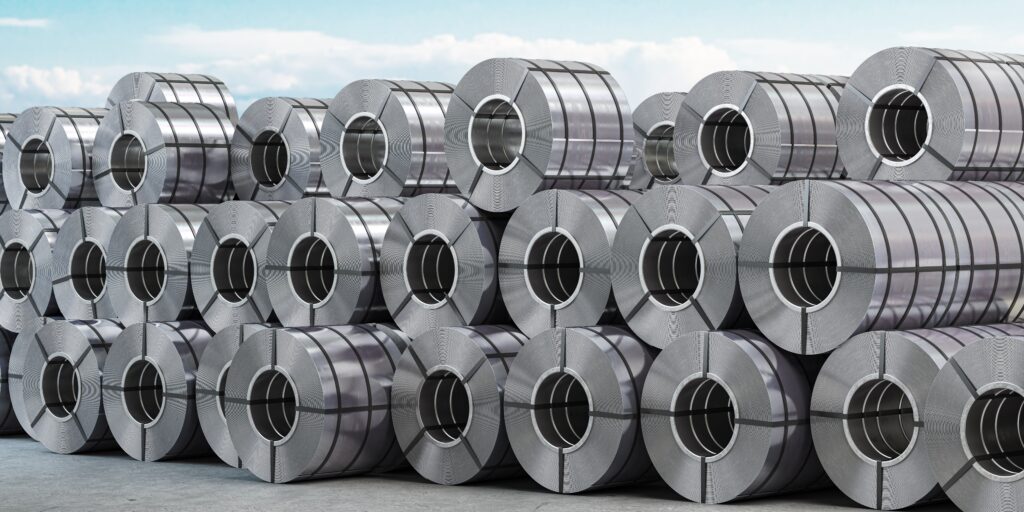
Inventory scores and metal futures returns
Inventory scores are quantamental (point-in-time) indicators of the inventory states and dynamics of economies or commodity sectors. Inventory scores plausibly predict base metal futures returns due to two effects. First, they influence the convenience yield of a metal and the discount at which futures are trading relative to physical stock. Second, they predict demand changes for restocking by producers and industrial consumers. Inventory scores are available for finished manufacturing goods and base metals themselves. An empirical analysis for 2000-2024 shows the strong predictive power of finished goods inventory scores and some modest additional predictive power of commodity-specific inventory scores.