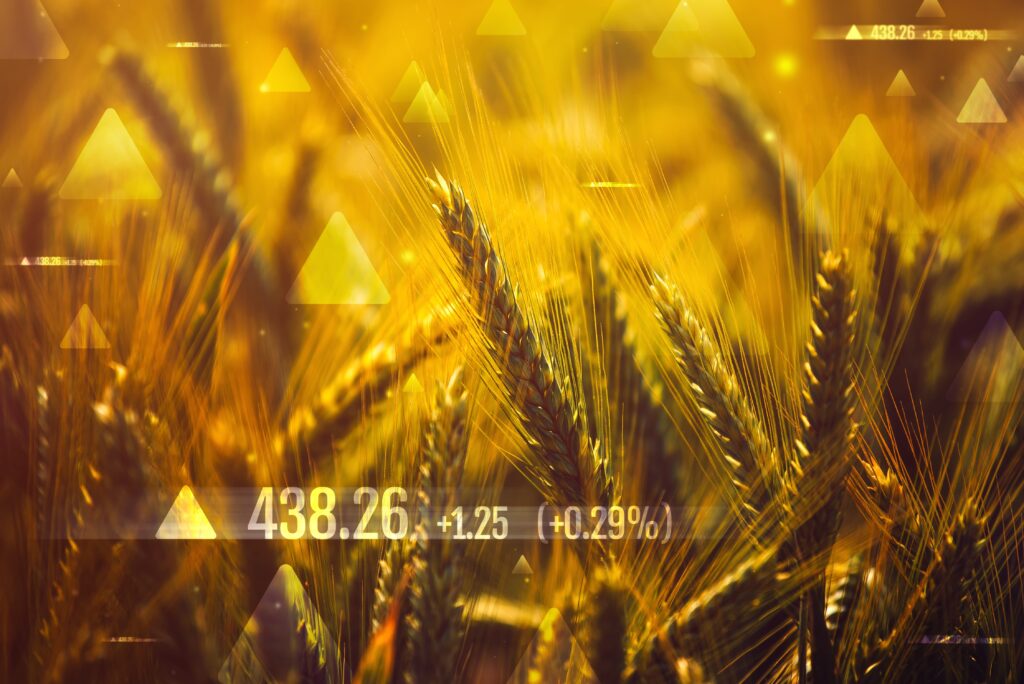
Commodity carry as a trading signal – part 1
Commodity futures carry is the annualized return that would arise if all prices remained unchanged. It reflects storage and funding costs, supply and demand imbalances, convenience yield, and hedging pressure. Convenience and hedging can give rise to an implicit subsidy, i.e., a non-standard risk premium, and make commodity carry a valid basis for a trading signal. An empirical analysis of carry for the front futures in 23 markets shows vast differences in size and volatility, with storage costs being a key differentiator. Also, carry is, on average, not strongly correlated across commodities, making it a more diversified signal contributor. To align carry measures more closely with expected premia, one can adjust for inflation, seasonal fluctuations, return volatility, and carry volatility. Most adjusted carry metrics display highly significant predictive power for returns.