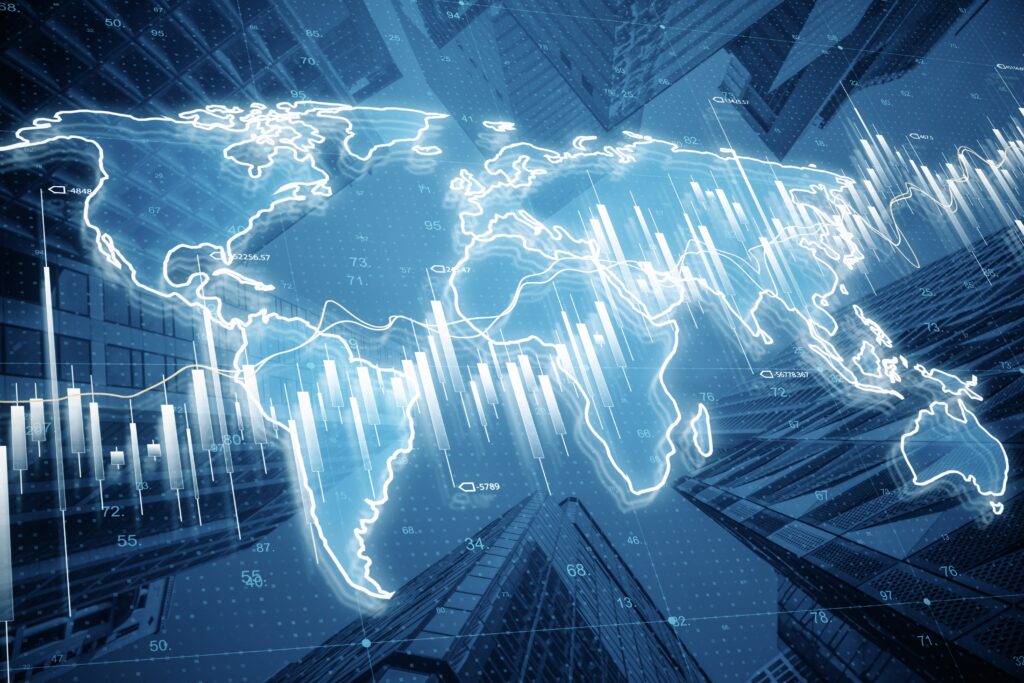
Boosting macro trading signals
Boosting is a machine learning ensemble method that combines the predictions of a chain of basic models, whereby each model seeks to address the shortcomings of the previous one. This post applies adaptive boosting (Adaboost) to trading signal optimisation. Signals are constructed with macro factors to guide positioning in a broad range of global FX forwards.
Boosting is beneficial for learning from a wide and heterogeneous set of markets over time, because it is well-suited for exploiting the diversity of experiences across countries and global economic states. Empirically, we generate machine learning-based signals that use regularized regression and random forest regression, and compare processes with and without adaptive boosting methods. For both regression types, machine learning prefers boosting as datasets get larger and, by doing so, creates more profitable signals.