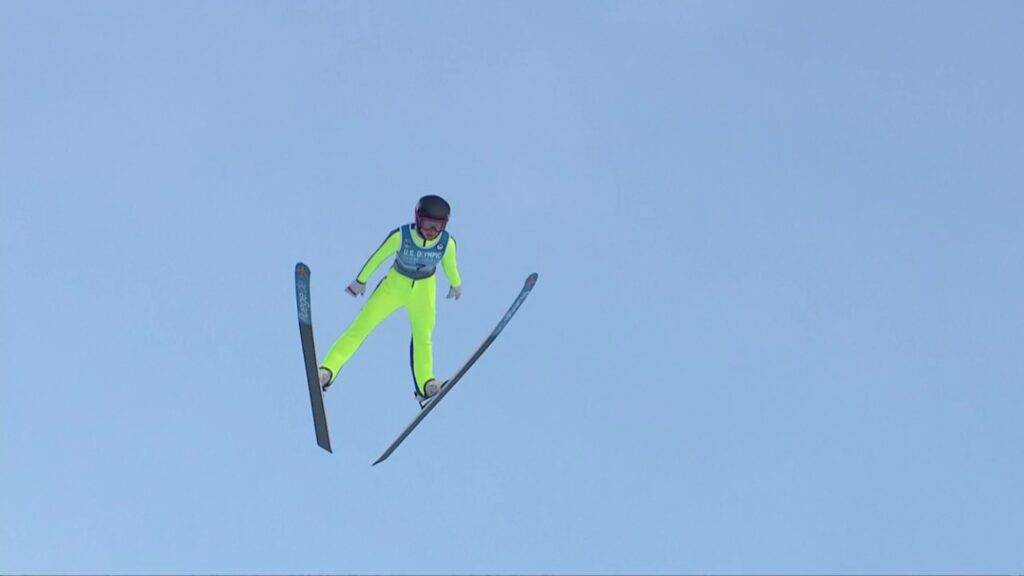
Equity convexity and gamma strategies
Equity convexity means that a stock outperforms in times of large upward or downward movements of the broad market: its elasticity to the market return is curved upward. Gamma is a measure of that convexity. All else equal, positive gamma is attractive, as a stock would outperform in market rallies and diversify in market stress. However, gamma is not observable, changeable, and needs to be estimated. Only a subset of stocks displays statistically significant gamma. Empirical analysis suggests that convex stocks can mostly be found in the materials, telecom, industrials, and energy sectors. High past volatility and price-to-book ratios have also been indicative of high gamma. Macroeconomic drivers that trigger gamma performance have been interest rates and oil prices. Systematic long-convexity strategies that seek to time convexity exposure have reportedly produced significant investor value.