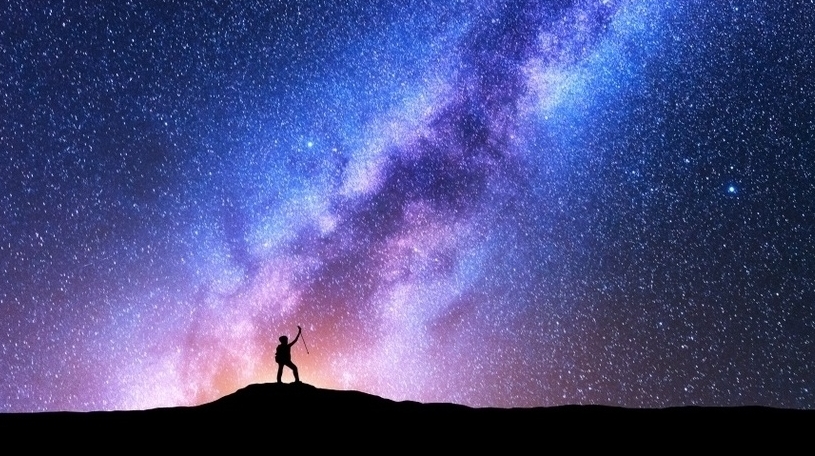
R tidyverse for macro trading research
The tidyverse is a collection of packages that facilitate data science with R. It is particularly powerful for macro trading research because [a] it supports efficient and standardized work with R’s vast universe of econometric models, [b] is well adapted for analyzing data vintages (i.e. data series that change over time), and [c] supports code in form of visually clean chains of statistical operations. The tidyverse’s core and peripheral packages share common design principles that harmonize workflow for crucial tasks: [1] organizing data structures, [2] transforming the content of data structures, [3] functional programming with complex nested data sets, [4] extraction of statistical information across models in a standardized form, [5] coding and mathematics with date-time objects, [6] coding with strings and regular expressions, [7] a flexible machine learning workflow, [8] highly versatile and consistent graphics creation, and [9] connectors to financial analysis packages.