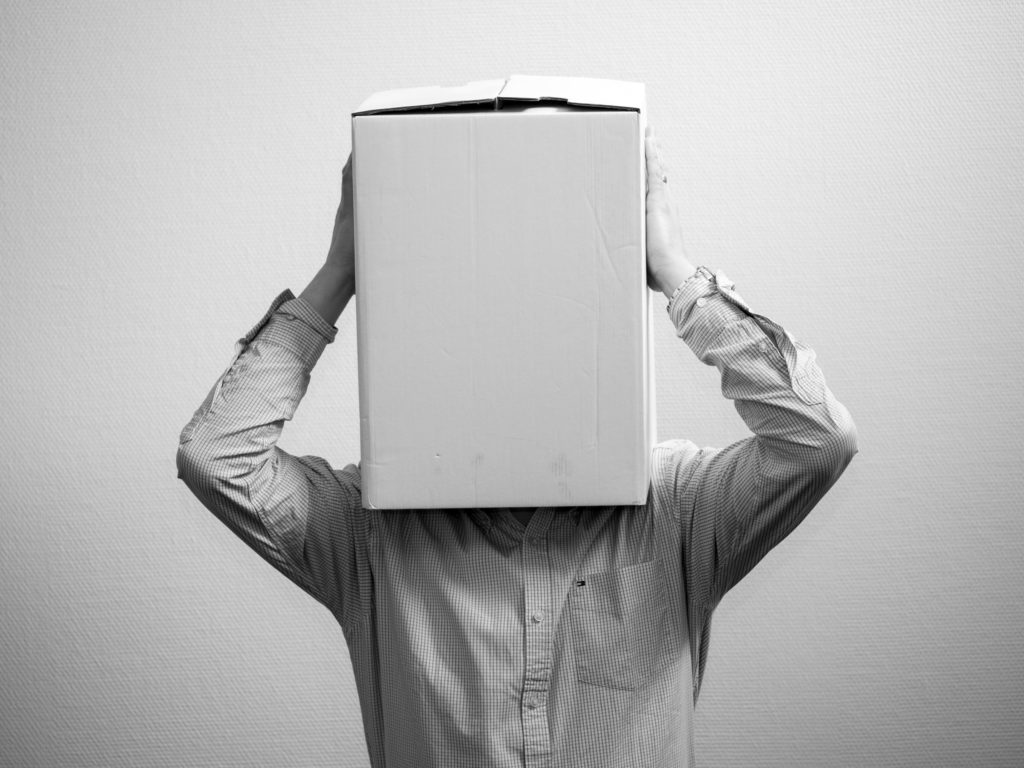
Markets’ neglect of macro news
Empirical evidence suggests that investors pay less attention to macroeconomic news when market sentiment is positive. Market responses to economic data surprises have historically been muted in high sentiment periods. Behavioral research supports the idea that investors prefer heuristic decision-making and neglect fundamental information in bullish markets, but pay more attention in turbulent times. This allows prices to diverge temporarily from fundamentals and undermines the conventional risk-return trade-off when sentiment is high. Low-risk portfolios tend to outperform subsequently. The sentiment bias also means that fundamental predictors of market prices work better in low-sentiment periods than in high-sentiment periods.