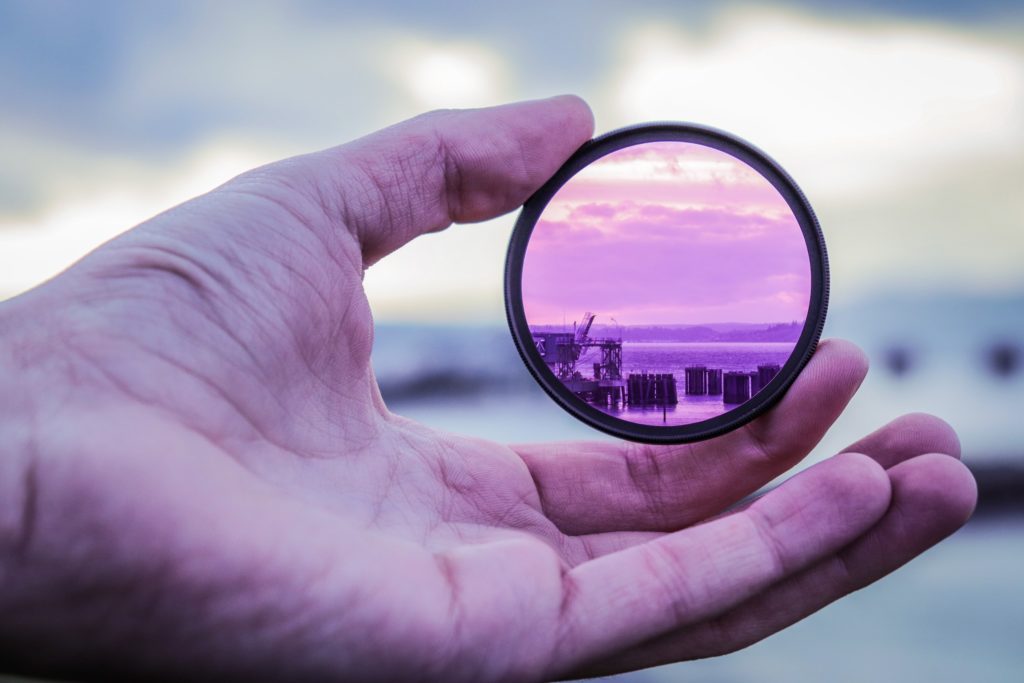
Ten things investors should know about nowcasting
Nowcasting in financial markets is mainly about forecasting forthcoming data reports, particularly GDP releases. However, nowcasting models are more versatile and can be used for a range of market-relevant information, including inflation, sentiment, weather, and harvest conditions. Nowcasting is about information efficiency and is particularly suitable for dealing with big messy data. The underlying models typically condense large datasets into a few underlying factors. They also tackle mixed frequencies of time series and missing data. The most popular model class for nowcasting is factor models: there are different categories of these that produce different results. One size does not fit all purposes. Also, factor models have competitors in the nowcasting space, such as Bayesian vector autoregression, MIDAS models and bridge regressions. The reason why investors should understand their nowcasting models is that they can do a lot more than just nowcasting: most models allow tracking latent trends, spotting significant changes in market conditions, and quantifying the relevance of different data releases.