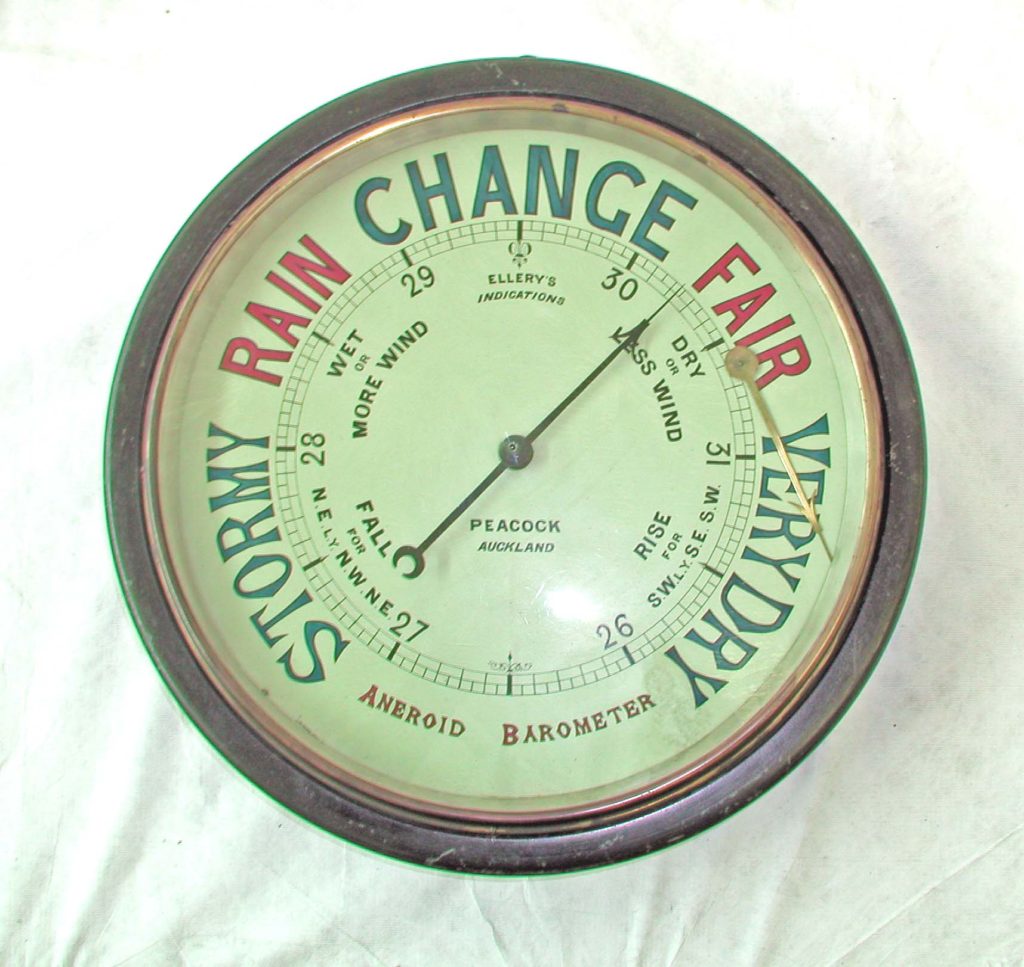
The dollar as barometer for credit market risk
The external value of the USD has become a key factor of U.S. and global credit conditions. This reflects the surge in global USD-denominated debt in conjunction with the growing importance of mutual funds as the ultimate source of loan financing. There is empirical evidence that USD strength has been correlated with credit tightening by U.S. banks. There is also evidence that this tightening arises from deteriorating secondary market conditions for U.S. corporate loans, which, in turn, are related to outflows of credit funds after USD appreciation. The outflows are a rational response to the negative balance sheet effect of a strong dollar on EM corporates in particular. One upshot is that the dollar exchange rate has become an important early indicator for credit market conditions.