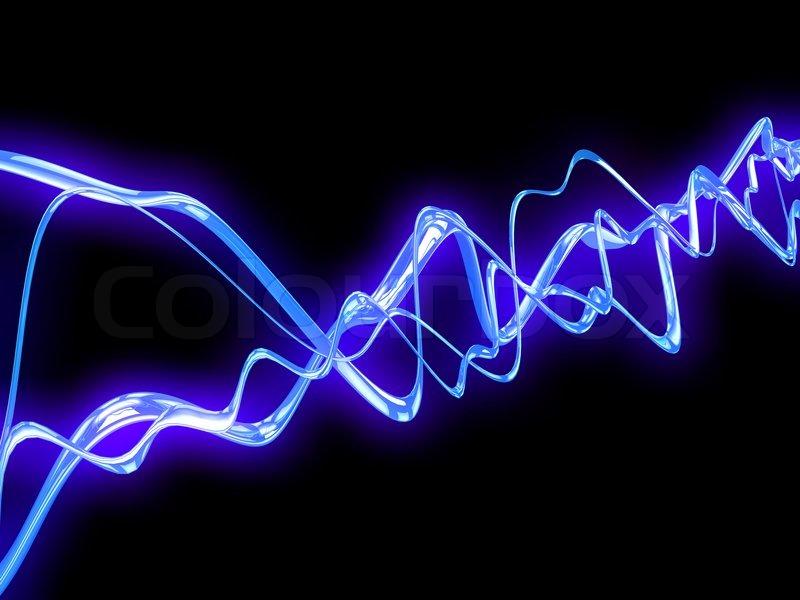
Nowcasting with MIDAS regressions
Nowcasting macro-financial indicators requires combining low-frequency and high-frequency time series. Mixed data sampling (MIDAS) regressions explain a low-frequency variable based on high-frequency variables and their lags. For instance, the dependent variable could be quarterly GDP and the explanatory variables could be monthly activity or daily market data. The most common MIDAS predictions rely on distributed lags of higher frequency regressors to avoid parameter proliferation. Analogously, reverse MIDAS models predict a high-frequency dependent variable based on low-frequency explanatory variables. Compared to state-space models (view post here), MIDAS simplifies specification and theory-based restrictions for nowcasting. The R package ‘midasr’ estimates models for multiple frequencies and weighting schemes. In practice, MIDAS has been used for nowcasting financial market volatility, GDP growth, inflation trends and fiscal trends.