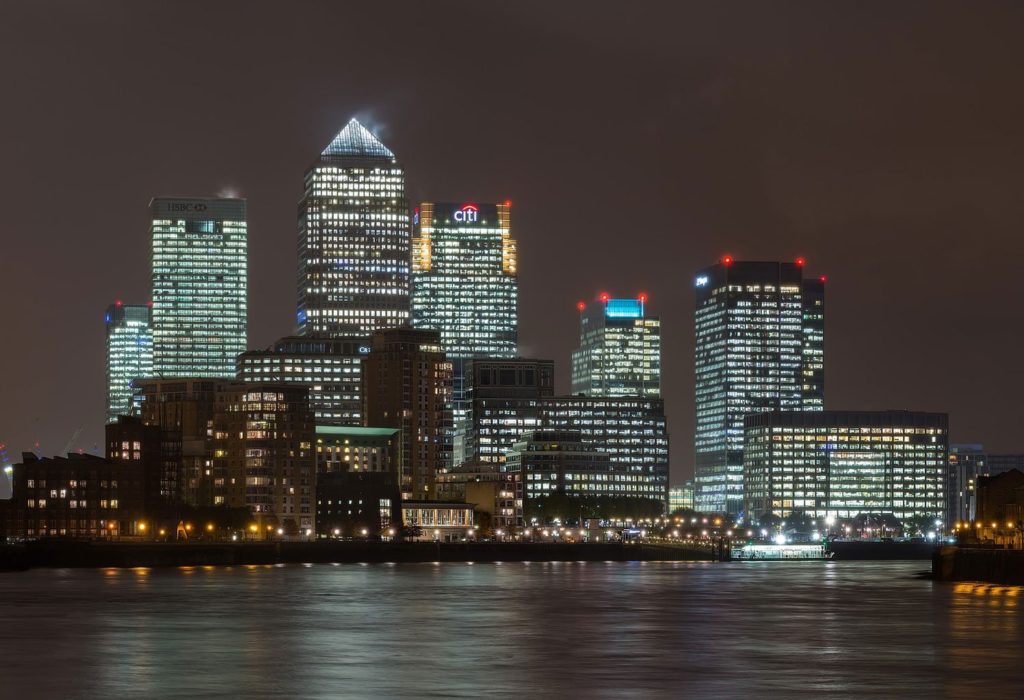
Dealer capital ratios and FX carry returns
When financial market intermediaries warehouse net risk positions of other market participants the marginal value of their capital should affect the expected and actual returns of such positions. This is of particular importance in the FX market, where excess positions typically end up on the balance sheets of a small group of international banks. Empirical evidence confirms that currency returns have been related to the dynamics of capital ratios of the largest dealers. Excess returns on FX carry trades can, to some extent, be interpreted as compensation for the balance sheet risk. Currencies that trade at a high forward discount have paid off poorly when intermediary capital ratios decreased.