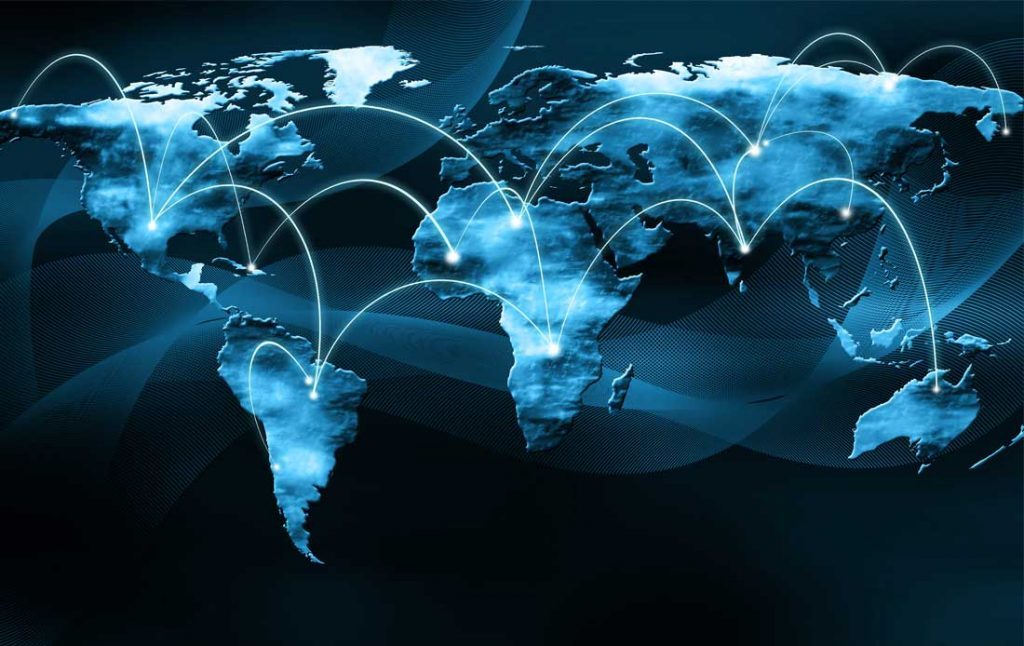
Macro trading and macroeconomic trend indicators
Macroeconomic trends are powerful asset return factors because they affect risk aversion and risk-neutral valuations of securities at the same time. The influence of macroeconomics appears to be strongest over longer horizons. A macro trend indicator can be defined as an updatable time series that represents a meaningful economic trend and that can be mapped to the performance of tradable assets or derivatives positions. It can be based on three complementary types of information: economic data, financial market data, and expert judgment. Economic data establish a direct link between investment and economic reality, market data inform on the state of financial markets and economic trends that are not (yet) incorporated in economic data, and expert judgment is critical for formulating stable theories and choosing the right data sets.