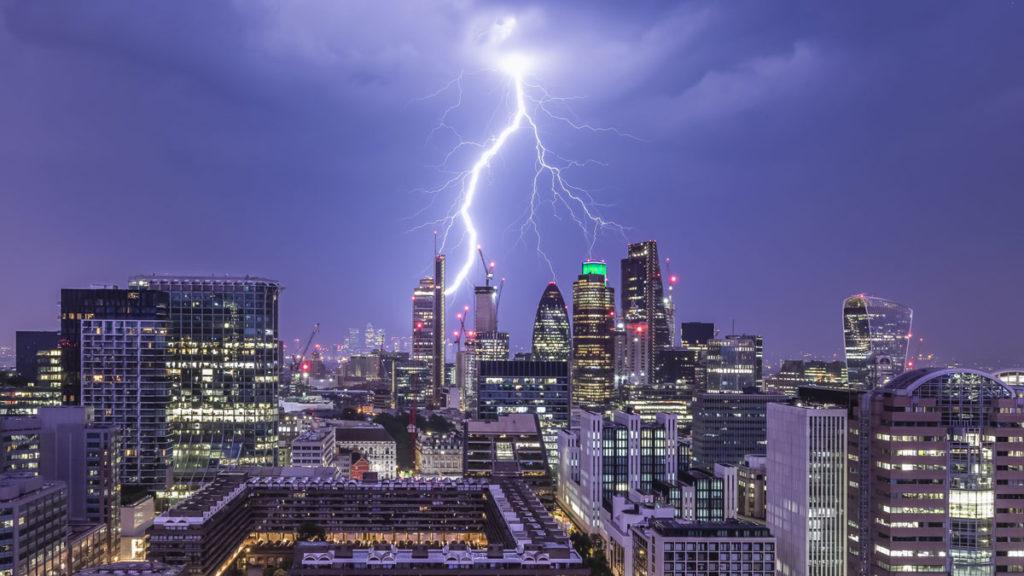
Modern financial system risk for macro trading
Financial system risk is the main constraint and disruptor of macro trading strategies. There are four key areas of modern systemic risk. [1] In the regulated banking sector vulnerability arises from high leverage and dependence on funding conditions. The regulatory reform of the 2010s has boosted capital ratios and liquidity safeguards. However, it has also induced new hazards, such as accumulation of sovereign risk, incentives for regulatory arbitrage, and risk concentration on central clearing counterparties. [2] Shadow banking summarizes financial intermediation outside the reach of standard regulation. It channels cash pools to the funding of asset holdings. Vulnerability arises from dependence on the market value of collateral and the absence of bank backstops. [3] Institutional asset management has grown rapidly in past decades and is now comparable in size to regulated banking. Asset managers play a vital role in global funding conditions but are prone to aggravating self-reinforcing market momentum. [4] Finally, emerging market financial systems have grown in size and complexity. China constitutes a global systemic risk factor due to the aggressive use of financial repression to sustain high levels of leverage and investment.