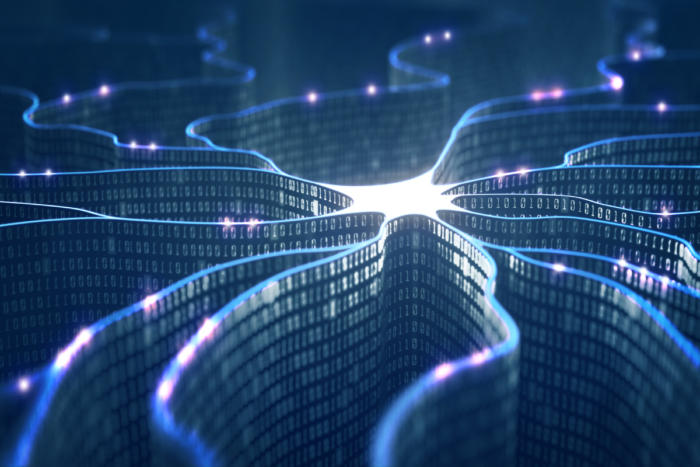
Modern backtesting with integrity
Machine learning offers powerful tools for backtesting trading strategies. However, its computational power and convenience can also be corrosive for financial investment due to its tendency to find temporary patterns while data samples for cross validation are limited. Machine learning produces valid backtests only when applied with sound principles. These should include [1] formulating a logical economic theory up front, [2] choosing sample data up front, [3] keeping the model simple and intuitive, [4] limiting try-outs when testing ideas, [5] accepting model decay overtime rather than ‘tweaking’ specifications, and [6] remaining realistic about reliability. The most important principle of all is integrity: aiming to produce good research rather than good backtests and to communicate statistical findings honestly rather than selling them.