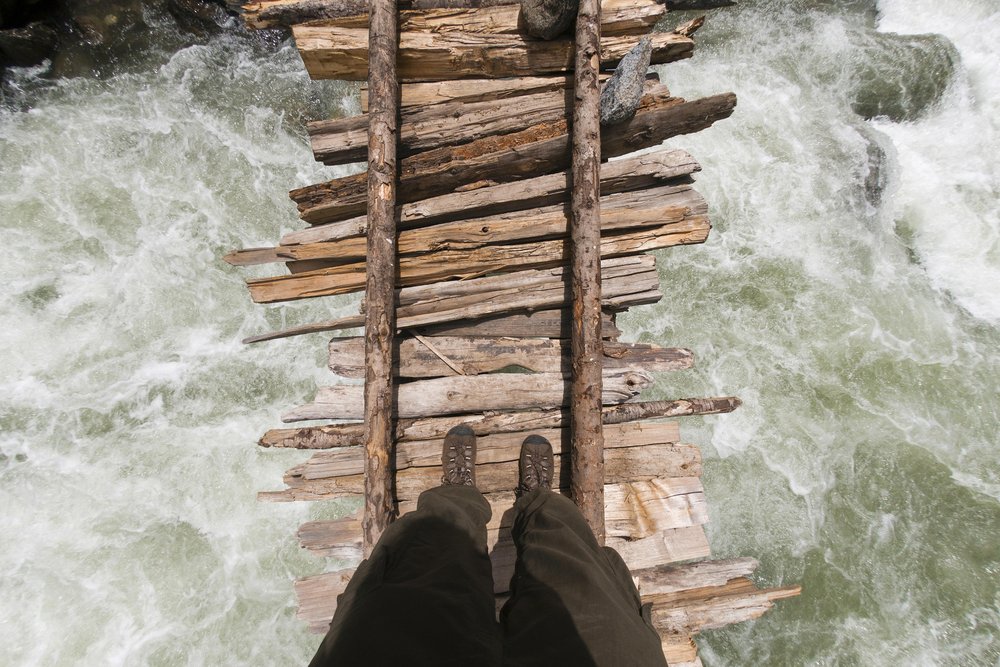
Bayesian Risk Forecasting
Portfolio risk forecasting is subject to great parameter uncertainty, particularly for longer forward horizons. This simply reflects that large drawdowns are observed only rarely, making it hard to estimate their ‘structural’ properties. Bayesian forecasting addresses parameter uncertainty directly when estimating risk metrics, such as Value-at-Risk or Expected Shortfall, which depend on highly uncertain tail parameters. Also, the Bayesian risk forecasting method can use ‘importance sampling’ for generating simulations that oversample the high-loss scenarios, increasing computational efficiency. Academic work claims that Bayesian methods also produce more accurate risk forecasts for short- and long-term horizons.